Predicting wheat yield gap and its determinants combining remote sensing, machine learning, and survey approaches in rainfed Mediterranean regions of Morocco
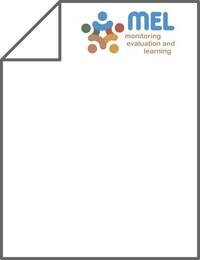
Authors:
Wheat plays a crucial role in Morocco’s food security, economic stability, and livelihoods of farming communities. Assessing key vegetation indices (as yield predictors), along with understanding potential yield, yield gap, and major determinants for this gap at regional and national scales, is vital for improving food security with resilience in variable climatic conditions. Analysing the yield gap and its causes during drought and optimal weather conditions can reduce crop failure risks and enhance productivity specially in variable rainfed production systems. This study aimed to develop scalable methodology to predict field- and landscape-level yield and yield gaps for wheat and their underlying causes examplifying Morocco’s rainfed production environment combining remote sensing, machine learning, and ground information. By analysing six vegetation indices (EVI2, CGVI, MSR, NDVI, OSAVI, and RVI) derived from Sentinel-2 satellite imagery (10 m resolution) over three successive growing seasons (2018–2019, 2019–2020, and 2020–2021), the study employed advanced vegetation index models for accurate prediction of wheat yields and yield gaps at plot and on a larger regional scale within the Rabat-Sale-Kenitra region. To identify the determinants of yield gap, climate and soil datasets were merged with crop management information and the random forest model was fine-tuned and assessed for each season and cumulatively. The findings highlighted that RVI, GCVI, and NDVI vegetation indices were particularly effective in predicting wheat yields, showing the highest R2 and the lowest prediction errors (RMSE). Such predictive methodologies are crucial for policymakers to proactively plan and mitigate risk minimization and adaption plans at regional and national levels. The models predicted rainfed potential yields of 5.99, 1.53, and 4.66 t ha−1, with corresponding yield gap of 3.38, 0.73, and 1.58 t ha−1 for the seasons of 2018/2019 (favorable); 2019/2020 (drought) and 2020/2021 (favorable), respectively. Across three periods, critical factors determining yield include soil moisture, total rainfall during the crop growing period, evapotranspiration, and soil texture and carbon content. To minimize drought risks and maximize benefits during variable rainfall conditions, it is essential to implement pre-season drought forecasts, customize seeding dates based on soil moisture, adopt technologies that enhance soil moisture retention, and utilize climate-adapted farming practices in the semi-arid and arid rainfed regions.